In this final part of our series, we continue our journey from the foundational discussions in Part 1 about Predictive Coding and the deep dive into Continuous Active Learning in Part 2. Here, we explore the cutting-edge potential of Large Language Models (LLMs) in eDiscovery. This post aims to illuminate how LLMs are set to revolutionize the field, offering solutions to the challenges previously discussed and introducing a new era of efficiency, scalability, and analytical depth in legal technology.
Autonomous Review Powered by LLMs
The legal world has always been an arena where accuracy, efficiency, and thoroughness are paramount. As the digital age has advanced, so has the volume of Electronically Stored Information (ESI) that requires Review in legal matters. Although revolutionary in their time, Traditional Technology-Assisted Review (TAR) methods are now being outpaced by the capabilities of Large Language Models (LLMs) in eDiscovery.
Here’s why:
- Holistic Understanding vs. Keyword Reliance—Traditional TAR methods often rely heavily on keyword searches. While effective to a degree, they are inherently limited in their ability to understand context, sentiment, or thematic nuances. On the other hand, LLMs can process vast amounts of information, not by merely latching onto specific words but by understanding the broader context, sentiment, and themes within the data. This ensures a comprehensive and nuanced review of ESI, which is vital in legal matters where the interpretation of a single document can sway the outcome of a case.
- End-to-End Solution vs. Fragmented Process—The eDiscovery Reference Model (EDRM) outlines the stages of managing ESI. While traditional TAR methods might be efficient in one or two stages, LLMs span the entirety of the eDiscovery process. From identifying pertinent documents to assisting in the final presentation of information, LLMs offer a holistic view and approach, making them an indispensable tool for legal professionals.
- Scalability and Evolution vs. Static Systems—As legal cases grow in complexity and the volume of ESI expands, there’s a dire need for systems that can adapt and scale. Traditional TAR methods might struggle with massive datasets or newer types of digital communications. However, LLMs are built to scale horizontally (handling more data types) and vertically (processing large datasets). This ensures that, regardless of the growth or evolution of digital data, LLMs remain robust and relevant.
Deep Analysis vs. Surface-Level Review—A cursory review of documents might overlook subtle yet crucial details. While TAR might identify keywords, LLMs go several layers deeper, understanding underlying themes, sentiments, and context. This ‘Deep Dive Approach’ ensures that legal professionals aren’t just skimming the surface but are equipped with a thorough understanding of their ESI.
Proactive Risk Management vs. Reactive Measures—One of the standout features of LLMs is their ability to anticipate issues, flag potential compliance violations, and identify data gaps that might jeopardize a case. Traditional methods might be more reactive, identifying problems after they’ve become evident. In contrast, LLMs offer a proactive approach, ensuring risk mitigation and compliance from the outset.
Cost-Effectiveness and Efficiency—The legal industry is aware that time is a precious commodity. LLMs significantly reduce the time spent on document review, translating to tangible cost savings. Furthermore, they allow human resources to be redirected to tasks that demand intricate human judgment, ensuring that professionals are used where they add the most value.
About the Author
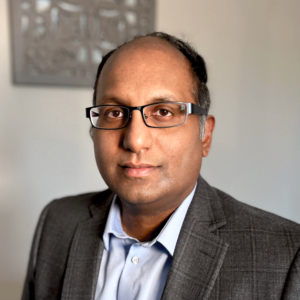
VASUDEVA MAHAVISHNU
Vasudeva is the CTO at Altumatim. Vasu brings his natural curiosity and passion for using technology to improve access to justice and our quality of life to the Altumatim team as he architects and builds out the future of discovery. Vasu blends computer science and data science expertise from computational genomics with published work ranging from gene mapping to developing probabilistic models for protein interactions in humans.